Promotion planning in general merchandize retail - Optimization challenges
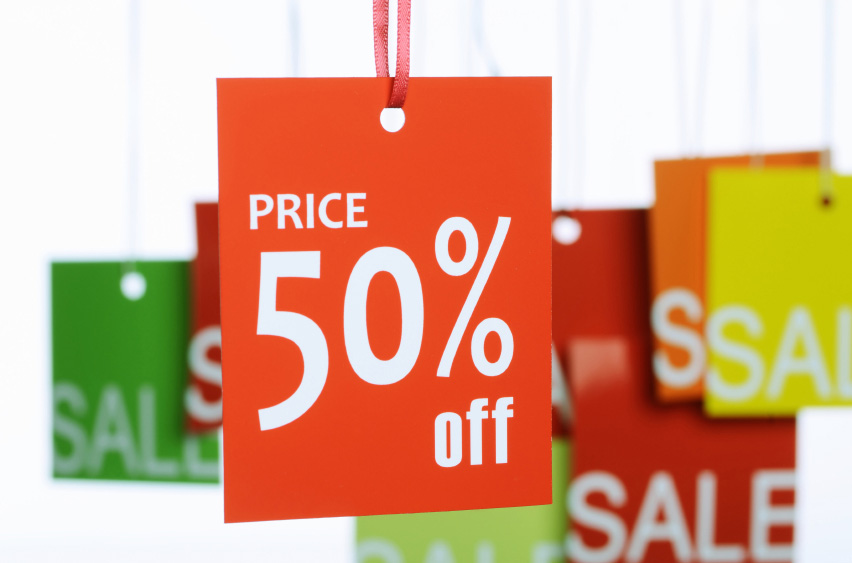
So far, we covered data challenges and process challenges in the context of promotional forecasts. In this post, the last of the series, we cover the very notion of quantitative optimization when considering promotions. Indeed, the choice of the methodological framework that is used to produce the promotion forecasts and measure their quantitative performance is critically important and yet usually (almost) completely dismissed.
As the old saying goes, there is no optimization without measurement. Yet, in case of promotions, what are you actually measuring?
Quantifying the performance of promotions
The most advance predictive statistics remain rather dumb in the sense that it’s nothing but the minimization of some mathematical error function. As a consequence, if the error function is not deeply aligned with the business, there is no improvement possible, because the measure of the improvement itself is off.
It doesn’t matter to be able to move faster as long you don’t even know if you’re moving in the right direction.
When it comes to promotions, it’s not just the plain usual inventory economic forces:
- inventory costs money; however, compared to permanent inventory, it can cost more money if the goods are not usually sold in the store, because any left-over after the end of the promotion will clutter the shelves.
- promotions are an opportunity to increase your market shares, but typically at the expense of the retailer’s margin; a key profitability driver is the stickiness of the impulse given to customers.
- promotions are negotiated rather than merely planned; a better negotiation with the supplier can yield more profits than a better planning.
All those forces need to be accounted for quantitatively; and here lies the great difficulty: nobody wants to be quantitatively responsible for a process as erratic and uncertain as promotions. Yet, without quantitative accountability, it’s unclear whether a given promotion creates any value, and if it does, what can be improved for the next round.
A quantitative assessment requires a somewhat holistic measure, starting with the negotiation with the supplier, and ending with the far reaching consequences of imperfect inventory allocation at the store level.
Toward risk analysis with quantiles
Holistic measurements, while being desirable, are typically out of reach for most retail organizations that rely on median forecasts to produce the promotion planning. Indeed, median forecasts are implicitly equivalent to minimizing the Mean Absolute Error (MAE), which without being wrong, remains the archetype of the metric strictly agnostic of all economic forces in presence.
But how could improving the MAE be wrong? As usual, statistics are deceptive. Let’s consider a relatively erratic promoted item to be sold in 100 stores. The stores are assumed to be similar, and the item has 1/3 chances of facing a demand of 6 units, and 2/3 of facing a demand of zero unit. The best median forecast is here zero units. Indeed, 2 units per store would not be the best median forecast, but the best mean forecasts, that is, the forecast that minimizes the MSE (Mean Square Error). Obviously, forecasting a zero demand across all stores is buggy. Here, this example illustrates how MAE can extensively mismatch business forces. MSE show similar dysfunctions in other situations. There is no free lunch, you can’t get a metric that is both ignorant of business and aligned with the business.
Quantile forecasts represent a first step in producing more reasonable results for promotion forecasts because it becomes possible to perform risk analysis, addressing questions such as:
- In the upper 90% best case, how many stores will face a stock-out before the end of the promotion?
- In the lower 10% worst case, how many stores will be left with more than 2 months of inventory?
The design of the promotion can be decomposed as a risk analysis, integrating economic forces, sitting on top of quantile forecasts. From a practical viewpoint, the method has the considerable advantage of preserving a forecast strictly decoupled from the risk analysis, with is an immense simplification as far the statistical analysis is concerned.
Couple both pricing and demand analysis
While a quantitative risk analysis already outperforms a plain median forecast, it remains relatively limited by design in its capacity to reflect the supplier negotiation forces.
Indeed, a retailer could be tempted to regenerate the promotion forecasts many time, varying the promotional conditions to reflect the scenarios negotiated with the supplier, however such a usage of the forecasting system would lead to significant overfitting.
Simply put, if a forecasting system is repeatedly used to seek the maximization of a function built on top of the forecasts, i.e. finding the best promotional plan considering the forecasted demand, then, the most extreme value produced by the system is very likely to be a statistical fluke.
Thus, instead the optimization process needs to be integrated into the system, analyzing at once both the demand elasticity and the supplier varying conditions, i.e. the bigger the deal, the more favorable the supplier conditions.
Obviously, designing such a system is vastly more complicated than plain median promotion forecasting system. However, not striving to implement such a system in any large retail network can be seen as a streetlight effect.
A policeman sees a drunk man searching for something under a streetlight and asks what the drunk has lost. He says he lost his keys and they both look under the streetlight together. After a few minutes the policeman asks if he is sure he lost them here, and the drunk replies, no, that he lost them in the park. The policeman asks why he is searching here, and the drunk replies, “this is where the light is”.
The packaged technology of Lokad offers limited support to handle promotions, but this is an area that we address extensively with several large retailers, albeit in a more ad hoc fashion. Don’t hesitate to contact us, we can help.