Forecasting 3.0 with Quantile Grids
Delivering better forecasts has always been the core focus for Lokad. Today, we are unveiling the third generation of our forecasting technology based on quantile grids. In layman’s terms, quantile grids demonstrate an unprecedented level of performance which means that your company can service more clients, more reliably, and with less inventory. Unlike all the existing forecasting methods available on the market, quantile grids do not provide one demand forecast per product, but provide the entire probability distribution for (nearly) all possible futures. Quantile Grids are made possible through the combination of Machine Learning, Big Data, Cloud Computing plus some commerce-driven insights.
Quantile Grids are now available in production for all our clients, accessible through a new Quantile Grid option for any inventory forecasting project.
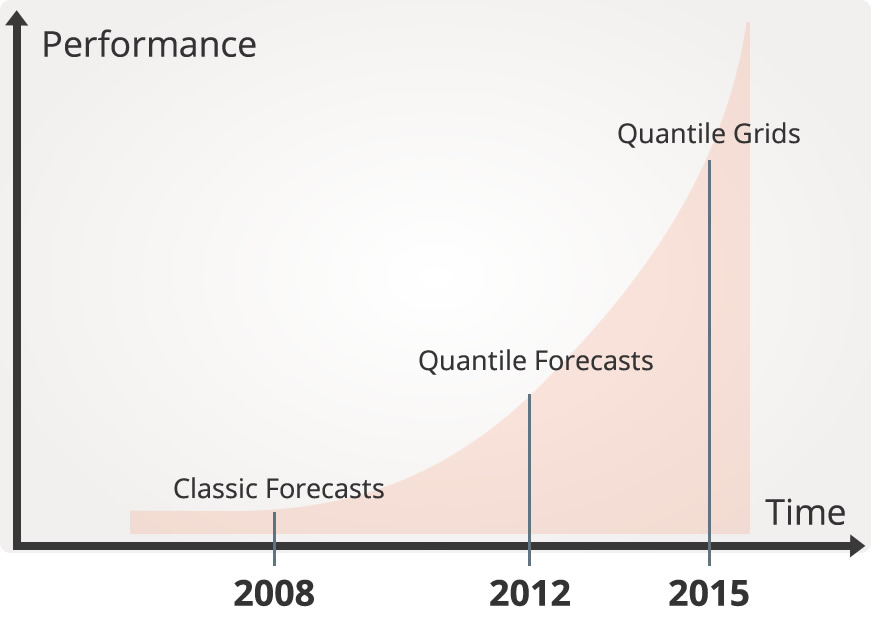
Forecasting 1.0: classic forecasts
When Lokad was founded back in 2008, we started with what we call now classic forecasts, our version 1.0, that is, a forecasting methodology where each product or SKU is associated with a periodic value; for example weekly forecasts of up to 13 weeks ahead. Implicitly, these forecasts are median forecasts: unbiased forecasts are expected to have 50% chances to be above or below the future demand. For the rest of the market, these forecasts are not referred to as the classic forecasts, they are the only forecasts because most of our competitors never even considered any alternatives.
However, as far commerce is concerned, no matter how accurate the classic forecasts, they work poorly in practice. Intuitively, classic forecasts are simply not looking at what really matters. The average or median demand is the easy and uninteresting case where everything goes according to plan. The tough cases however are concerned with unexpectedly high or unexpectedly low demand because they respectively create stock-outs and dead inventory. These types of extreme situations are the ones that really cost money. Classic forecasts work poorly, not because the algorithms are not good, but because they do not look at the business from the correct angle. Thus, no matter how much R&D investment a company can put into classic forecasts, it just fails. This was one of the toughest lessons for Lokad to learn in our early days.
Forecasting 2.0: quantile forecasts
In 2012, we made our first breakthrough with quantile forecasts. Despite a name that might sound downright scary, quantile forecasts are something that is much closer to what executives are doing in for their companies: they are scenarized forecasts. Instead of looking at the average case, quantile forecasts have the following objective: let’s look at the Top 5% of our most optimistic demand prospects, will we suffer from a stock-out? Then, let’s look at the Worst 5% of our most pessimistic demand prospects, will we have to deal with dead inventory? Quantile forecasts directly tackle the tough questions that actually matter from a business perspective. As engineers say, it’s better to be approximately correct than to be exactly wrong, and while quantile forecasts also suffer from all the inaccuracies associated with the classic forecasts, quantile forecasts massively outperform classic forecasts from an operational perspective whenever inventory is involved.
Yet, quantile forecasts are not the pinnacle of forecasting either. On the surface, our quantile forecasting technology was suffering from numerical oddities such as quantile crossing and quantile instabilities. However, since those oddities are quite visible, they can be efficiently mitigated. However, on a deeper level, we realized that our quantile forecasts were still not perfectly aligned with the actual business tough spots. In particular, quantile forecasts are leaving the burden of optimizing the service levels to the Supply Chain Manager. This is cheating - in a way - because a considerable part of inventory performance is actually delivered via a very precise tuning of the most profitable service levels that adequately balance inventory costs and quality of service.
Forecasting 3.0: quantile grids
In February 2015, we are now releasing our second forecasting breakthrough: quantile grids. Over the years, we came to terms with the fact that forecasts can be nothing but imperfect. Accurate forecasts are a fairytale, conveniently repeated within a market overrun by underwhelming vendors. Since we cannot predict the exact future, what about trying to assign a probability to every single possible future? That is, the probability of selling zero units, one unit, two units, etc. This is exactly what quantile grids are about: delivering not only one forecast per product, but delivering the entire probability distribution of demand for every product. Under the hood, quantile grids are a little bit like quantile forecasts, except that a demand forecast is simultaneously computed at all service levels.
Optimizing inventory or managing supply chain is all about balancing risks and opportunities: inventory levels vs service levels, purchase price vs supplier lead time, bulk purchase vs made to order, and so on. While quantile forecasts can pinpoint one or two troublesome scenarios, in the end, it is just one forecast value per product, and no matter how good this value can be, this one value cannot capture all the diversity of possible business outcomes. In contrast, quantile grids tackle the problem head-on: all outcomes are computed and associated with their respective probabilities. For every scenario, such as for the future demand of 3 units, we have bought only 2 units, it then becomes possible and straightforward to compute the net business result – like 2 units sold, and 1 unit missed. As a result, every purchase decision can be assessed by simply unrolling all the scenarios and applying the calculated probability to each scenario.
A breakthrough coming from aerospace
While Lokad primarily services retailers, we do such other industries as well, such as aerospace. One year ago, we started working for a large joint venture between AirFrance Industries and Lufthansa Technik, and realized that our quantile forecasting technology was not entirely up to the challenge. Each quantile forecast is like a single business scenario. While it is possible to combine 3, 4 or 5 different business scenarios, it takes great effort to implement the rules that glue all of these scenarios together in order to produce optimized supply decisions.
A much more elegant solution, and one which also yields much better inventory performance, consists of forecasting and evaluating all future business scenarios. No more ad-hoc scenarios that we desperately try to weld together, but a listing of (nearly) all possible scenarios (granted, it is a long listing), all treated in a simple and uniform way. This approach comes with the downside of being brutally more demanding as far computing resources are concerned. However, thanks to our favorite cloud computing platform - Microsoft Azure - computing resources have never been cheaper, and prices are still in free fall.
The results that we obtained through quantile grids for aerospace proved to be dwarfing the performance of our flagship quantile forecasting technology. It was time to bring back the rocket science (well, not rockets, jet airliners actually) to merchants, and the multiple experiments that we had performed over the last couple of months confirmed the decisive superiority of quantile grids compared to our original quantile forecasts.
Future of predictive commerce optimization
When we first released quantile forecasts three years ago, I predicted that within 10 years, quantile forecasts would be the default tool for any supply chain practitioner serious about her inventory performance. Well, it turned out that the efforts of the entire Lokad team, including my own, proved me wrong. As we have uncovered an approach superior to our initial quantile forecasts, we came to the conclusion that the long-term future of quantile forecasting is brittle. Yet, the future of the descendant of quantile forecasts is brighter than ever, as quantile grids solve the challenges that had been eluding us for years, such as the optimization of service levels, container shipments or multi-sourcing strategies.
Also, for years, inventory forecasting and pricing optimization have been treated in strict isolation, as if they were parts of two separate puzzles: the demand forecasting engine ignored what happens on the pricing side, and to make them even, the pricing engine did not care about the supply chain constraints either. However, stocks and prices are two sides of the same coin; and we now realize that any optimization attempt that blindly ignores the other side of the coin is a naive attempt at best.
Thus, while I will avoid making the same mistake and predicting that quantile grids are the long-term future of forecasting only to be proved wrong by Lokad’s team later on, I will now more safely bet that whatever predictive technology emerges from our efforts, pricing analysis will probably become unified with stock analysis along the way. We are not quite there yet, but we are making steady progress in this direction.
New methodology: purchasing prioritization
All inventory optimization systems (Lokad 2.0 included) compute reorder points. By comparing reorder points with quantities on hand and on order, these systems also compute suggested reorder quantities. Over the years, we have discovered two major limitations of this approach. First, those systems do not say anything about the target service levels, and their optimization. Second, reorder points prove to be somewhat inflexible whenever purchase constraints are involved.
Inventory optimization systems traditionally produce a static set of reorder points (one per SKU), primarily driven by their respective user-defined service levels. However, this is cheating because the burden of figuring out the “optimal” service level falls back to supply chain planner; and not only does figuring out the correct service levels proves to be a very time-consuming exercise, it is also a source of major inefficiencies if the service levels are inadequately chosen.
With quantile grids, the picture is very different: a master purchase priority list is calculated. Technically, it is a list where each SKU appears on numerous lines, each line being associated with a suggested order quantity – typically 1 unit if no supply constraints are present. The list is prioritized, and this prioritization criterion is of primary importance.
For most businesses, this prioritization answers the question: for $1 of extra inventory what is the next unit which gives the company the highest returns? This can also be formulated as the expected gross margin minus the expected inventory carrying costs. Naturally, as we go through down the list, the expected gross margin sharply decreases, because the probability of having a demand sufficiently high to absorb the stock becomes very thin. Similarly, when going down the list, the inventory carrying cost sharply increases, as each extra unit of inventory is expected to remain in the warehouse for longer. In theory, the list has no end, as it goes down to infinity. In practice however, we simply stop at a point well beyond what would constitute “reasonable” inventory levels. When a purchase is made, the goal is not to go down the list but to buy items according to their respective priorities, and to stop buying once the spending target is reached.
Consequently, this entirely removes the need to specify the service levels. Once a spending budget is defined, a company purchases their goods based on the priorities established by the master purchase priority list. Purchasing goods in this order ensures that the company’s revenues or profits are maximized, following the specified prioritization criteria.
Quantile grids are also much more versatile in their capacity to address scenarios that involve supply constraints. While quantile forecasts are indeed powerful, as soon as you have minimal order quantities, either per SKU or per supplier, and possibly some container volume capacity constraints too, the suggested quantities do not match the supply constraints. And it is then up to the supply chain planner to deal with all the adjustments, namely removing certain SKUs or increasing the units for other SKUs, in order to compose a complex order batch that fulfills all the constraints.
With quantile grids, we have a much more compelling and a much more straightforward user experience to propose. The master list makes it simple to accommodate ordering constraints. If minimal ordering quantities per SKU are present, then, the ineligible lines can be removed from the list. Similarly, if a target capacity constraint exists to accommodate container shipments, then purchase entries can be processed in following the order of the list until the target capacity is reached.
What’s next?
While quantile grids are already live and accessible to all companies who have an open Lokad account, we are still lacking documentation that outlines both the technical aspects, but also the supply chain best practices relating to this new technology. This material is coming. Stay tuned.